As we forge ahead into an era of increased digitization, the prowess of Conversational AI is becoming a hot topic. From chatbots to virtual assistants, interactive dialogue systems play an essential role in numerous sectors. At the heart of these systems are sophisticated language models. Today, we’re focusing on one of the most powerful tools out there, ChatGPT, and ChatGpt optimizing language models for dialogue.
Understanding ChatGPT
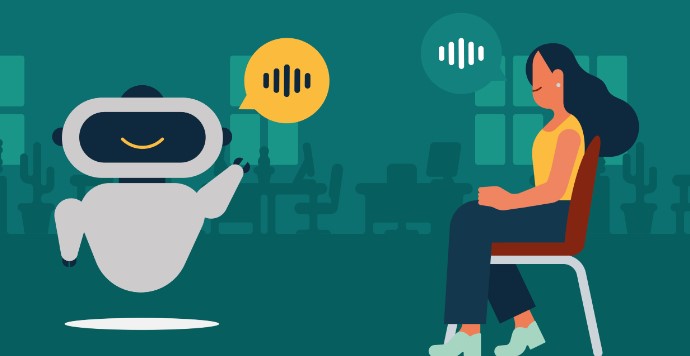
ChatGPT, an initiative by OpenAI, represents the pinnacle of natural language processing (NLP) and language generation. It is an advanced language model capable of generating human-like text responses, making it a key player in the realm of text-based conversational interfaces.
The goal of ChatGPT is to facilitate and improve human-computer interaction by producing coherent and context-aware responses. However, making a chatbot understand and generate conversation as a human would is no small task. This brings us to the complex, yet intriguing process of optimizing language models for dialogue systems.
Optimizing Language Models for Dialogue
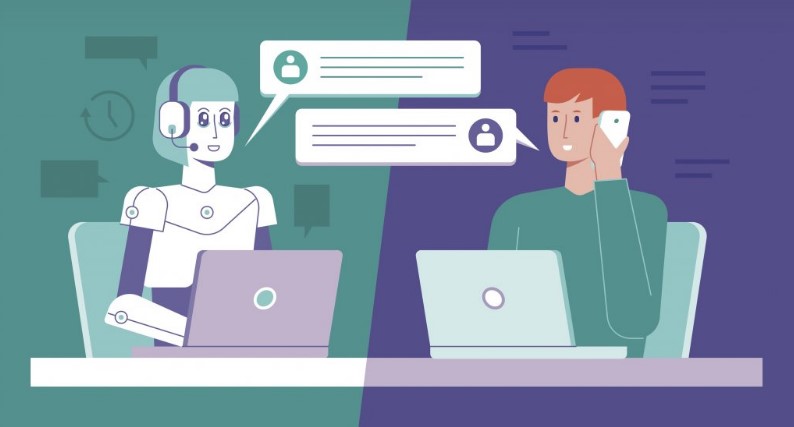
Dialogue optimization is crucial in creating chatbots that can deliver satisfying user experiences. This involves honing the system’s ability to understand the intricacies of human conversation, including semantics, sentiment, and context. So, how does dialogue optimization work with ChatGPT?
The optimization process incorporates a two-step approach: pre-training and fine-tuning. During pre-training, the model learns to predict the next word in a sentence, ingesting vast amounts of text data. This step enables the model to learn grammar, facts about the world, and some reasoning abilities. The fine-tuning phase, on the other hand, refines the model using specific dialogue datasets and human feedback, optimizing the model for interaction.
Role of NLP in Dialogue Systems
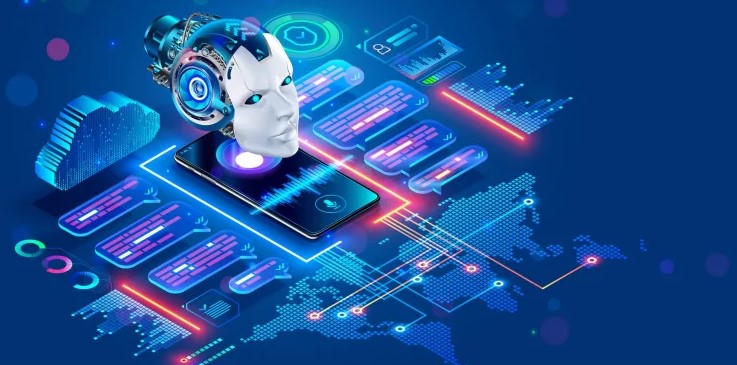
NLP plays an integral role in the optimization process. By analyzing and understanding human language, NLP allows ChatGPT to recognize the context, interpret the meaning, and generate relevant responses. It also incorporates dialogue management principles, which help the model maintain a coherent conversation with the user by keeping track of the dialogue history.
Context-aware Responses
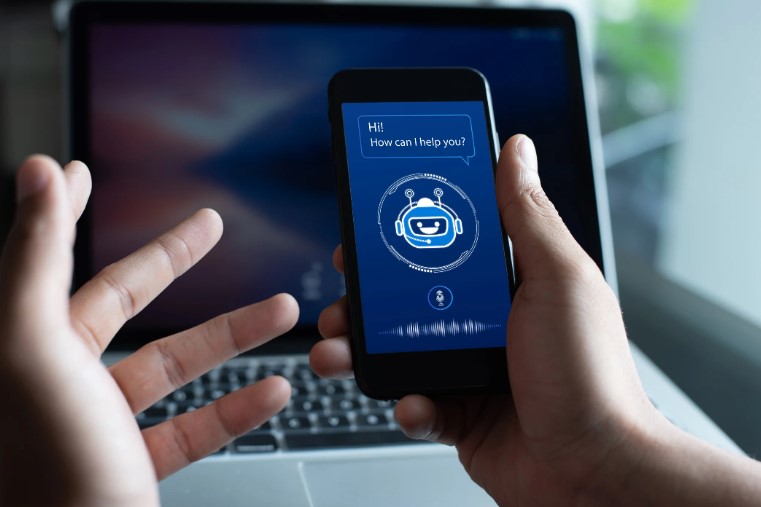
A significant challenge in optimizing language models for dialogue is enabling the system to understand and respond according to the context. The implementation of context-aware responses in ChatGPT has significantly improved its ability to maintain meaningful interactions. Using advanced algorithms, the model analyzes the current conversation thread to provide relevant responses, making the conversation flow more naturally.
Sentiment Analysis
Sentiment analysis in dialogue systems adds another layer of sophistication to language models. By analyzing the user’s sentiment, ChatGPT can adjust its responses to match the tone and context of the dialogue better. This enhances the user experience by making interactions feel more human-like, leading to improved user satisfaction.
Are There Ongoing Developments in Optimizing Language Models?
Absolutely! The field of conversational AI is constantly evolving. Current research and developments are geared towards making language models like ChatGPT more efficient, context-aware, and user-friendly. As we move forward, we can expect these advancements to revolutionize how we interact with technology, bringing us one step closer to seamless human-computer interaction.
Machine Learning and Conversational Agents
The power of machine learning is central to the effectiveness of modern conversational agents like ChatGPT. Through machine learning, these language models can “learn” from previous interactions and improve over time. The more data the model interacts with, the more efficient and accurate its responses become.
There are several machine learning strategies deployed in the optimization of language models for dialogue. Deep learning algorithms, which are a subset of machine learning, are particularly beneficial. They allow the model to analyze complex patterns in large datasets, significantly improving its comprehension and generation capabilities.
Reinforcement Learning
Reinforcement learning is another key approach in optimizing language models for dialogue. This strategy relies on a system of rewards and punishments to train the model. For example, if ChatGPT provides an appropriate response during training, it’s rewarded. If the response is inadequate or incorrect, it receives a penalty.
By applying reinforcement learning, the model can progressively improve, refining its ability to generate context-appropriate responses. This approach is especially beneficial in managing long dialogue chains and maintaining coherence in ongoing conversations.
Advanced Dialogue Management
Dialogue management is a critical aspect of conversational AI, dictating how a system interacts with the user. For instance, it helps the model determine when to request more information, when to change the topic, or when to conclude the dialogue. In optimizing ChatGPT, dialogue management strategies are continually refined to ensure smoother, more engaging conversations.
A central part of dialogue management is the dialogue state tracker, which keeps track of the conversation’s progress. It enables the system to manage multiple conversational threads and ensure continuity in interactions, thereby leading to more satisfactory user experiences.
Impact of Semantic Understanding in Dialogue Systems
While much of the focus in language model optimization is on syntax — the structure of language — understanding semantics, or the meaning of words and sentences, is equally crucial. Semantic understanding allows ChatGPT to comprehend the subtleties of human language, like idioms, cultural references, and sarcasm.
Incorporating advanced semantic understanding algorithms in ChatGPT has significantly improved its ability to generate more nuanced and contextually apt responses, thereby enhancing the overall conversational experience for users.
Future Outlook
As we venture further into the field of conversational AI, new advancements promise to revolutionize how we optimize language models for dialogue. One such promising development is the use of transformer models, which offer improved context understanding and response generation.
Moreover, the field of affective computing, which involves the development of systems and devices that can recognize and interpret human emotions, holds potential for further enhancing the human-like interaction capabilities of ChatGPT.
As research and development continue in this fascinating domain, we can look forward to a future where conversational agents like ChatGPT communicate more effectively, understand us better, and provide even more value in our daily lives.
FAQs
How Can Language Models Be Optimized for Dialogue Systems?
Optimizing language models for dialogue systems involves various techniques, including NLP, dialogue management, sentiment analysis, and context-aware responses. These techniques help the system understand and respond to human language more effectively.
What Role Does Natural Language Processing Play in Optimizing Dialogue Systems?
NLP is a fundamental component in optimizing dialogue systems. It allows the system to interpret and generate human language, thereby enabling it to communicate effectively with users.
Are There Any Specific Algorithms for Optimizing Language Models in Conversational Agents?
Several algorithms can be employed in optimizing language models for conversational agents. The choice of algorithm largely depends on the specific requirements of the system, including the complexity of the dialogue and the need for context-aware responses.
What Are the Challenges and Solutions for Optimizing Language Models for Interactive Dialogue Systems?
Some challenges in optimizing language models include maintaining context in dialogues, handling ambiguous user input, and managing the diversity of human language. Solutions include implementing advanced NLP techniques, developing context-aware algorithms, and incorporating sentiment analysis.
How Does Machine Learning Contribute to Language Model Optimization?
Machine learning plays a vital role in language model optimization. It allows the model to learn from previous interactions and progressively improve its response generation capabilities. This learning process involves algorithms that can analyze complex patterns in large datasets, thereby enhancing the model’s understanding of human language.
What Is the Role of Reinforcement Learning in Optimizing Language Models?
Reinforcement learning is a strategy used to train language models. It involves a system of rewards and penalties that encourage the model to generate appropriate responses. Over time, this learning process improves the model’s ability to maintain coherence and context in ongoing dialogues.
How Does Semantic Understanding Enhance Dialogue Systems?
Semantic understanding allows a dialogue system to comprehend the meaning of words and sentences. This ability enhances the system’s response capabilities, enabling it to generate nuanced and contextually appropriate responses. It helps the system understand subtleties like idioms, cultural references, and sarcasm, which leads to a more human-like conversational experience.
Also Read: Assimilation AP Human Geography: A Comprehensive Guide
Conclusion
To sum up, the process of “ChatGpt optimizing language models for dialogue” is intricate yet rewarding. With tools like ChatGPT, we are continuously pushing the boundaries of what’s possible in conversational AI. This journey of innovation and improvement holds the promise of an exciting future where technology communicates as effortlessly as a human companion. So, let’s look forward to a world where our interactions with AI are more natural, engaging, and fruitful than ever before.
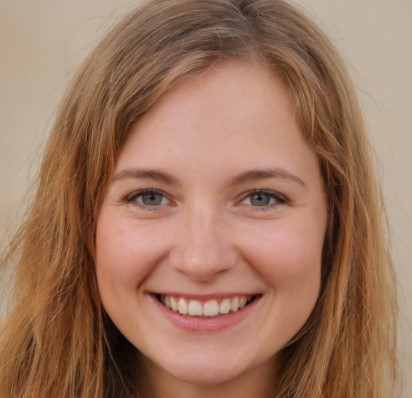
Meghan Wiltshire, the captivating blogger and wordsmith extraordinaire. With her pen as her wand, she weaves enchanting tales and imparts wisdom through her engaging writing. Join her on a literary journey that will leave you spellbound and craving for more.